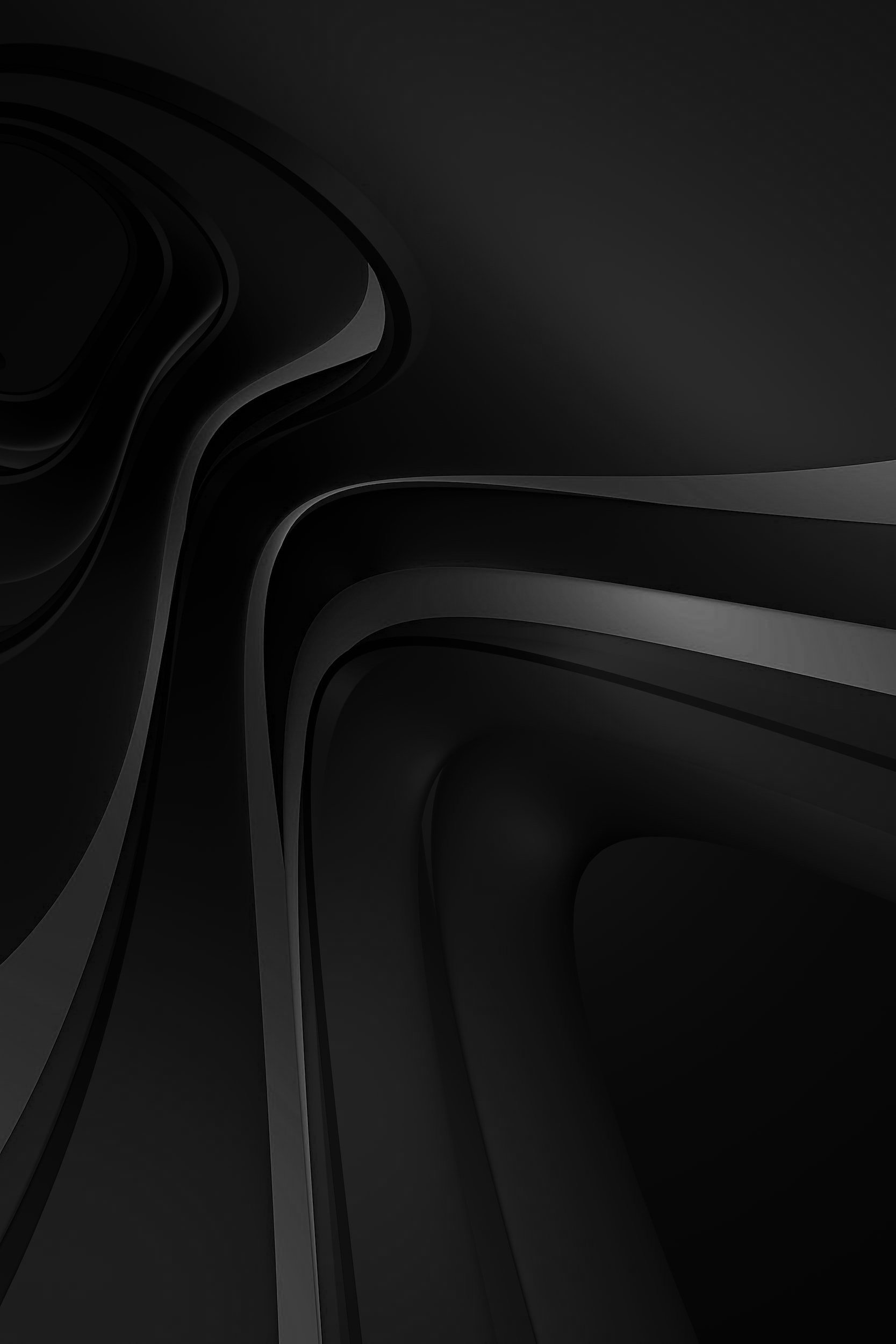
We believe enabling broader participation and open science is key to increase transparency and reduce potential harms from emerging AI technologies.
EleutherAI has trained and released several series of LLMs and the codebases used to train them. Several of these LLMs were the largest or most capable LLMs available at the time, and have been widely used since in open-source research applications. We believe that open access to these technologies is crucial to empower independent research.
One of our core research directions is interpretability: understanding how and why AI systems behave. Interpretability is vital to understand how to predict or modify model behavior and to ensure that systems are optimizing the metrics that we desire, so that these systems can be trusted.
Current machine learning systems are black boxes built to minimize metrics and objectives that need not reflect the desires of their developers or users, especially when deployed in critical decision-making scenarios. It is therefore vital before the fact to understand how to eliminate these failures and develop systems that more robustly behave as intended.
Our main research focus is on language models, but we additionally perform research spanning other modalities, including image and audio data.